Enhancing Urban Resilience Against Liquefaction Through Machine Learning
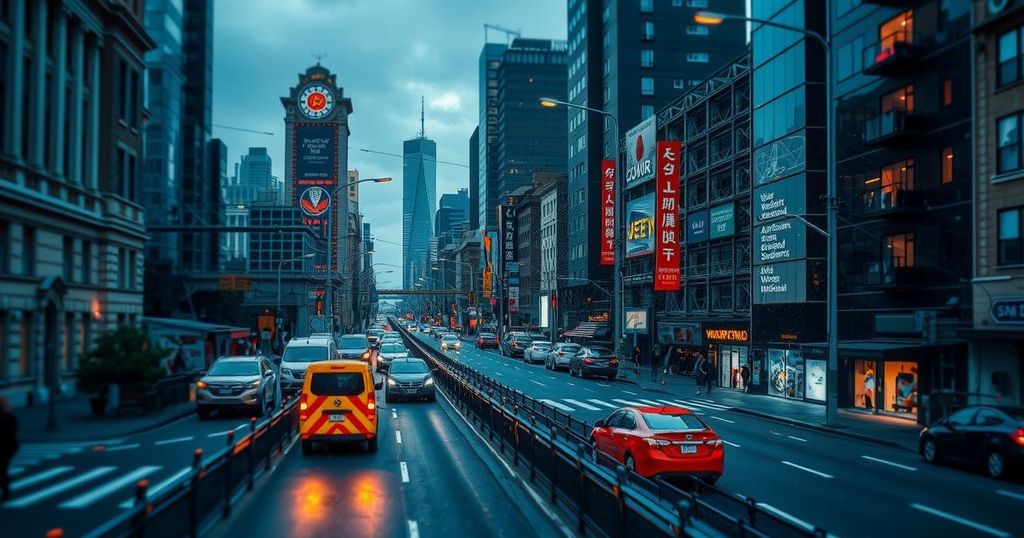
Researchers from Japan have developed a machine learning model that produces contour maps of soil bearing layers, aiding in the prediction of liquefaction risk in earthquake-prone areas. Their approach utilizes artificial neural networks and data from 433 locations in Setagaya, Tokyo, enhancing disaster planning and construction site selection. Historical liquefaction events underscore the model’s importance in urban resilience strategies.
In an effort to enhance urban resilience against liquefaction, researchers from Shibaura Institute of Technology in Japan have developed a novel machine learning model capable of generating detailed contour maps that display the depth of soil bearing layers. This advancement enables city planners to pinpoint areas that are particularly susceptible to liquefaction, a significant risk in earthquake-prone regions such as Japan. Utilizing artificial neural networks (ANNs) and ensemble learning techniques, the team created 3D maps based on data collected from 433 locations in Setagaya, Tokyo. The phenomenon of liquefaction, where loosely packed water-saturated soils behave as a liquid due to intense shaking, poses severe threats to infrastructure during earthquakes. Historical data illustrates the substantial damage inflicted by liquefaction, including the devastation caused by the 2011 Tōhoku earthquake, which resulted in harm to over a thousand homes, alongside the destruction of essential utilities in Christchurch and during the recent Noto earthquake that impacted more than 6,700 residences. Professor Shinya Inazumi and his student Yuxin Cong aim to address these critical challenges by implementing machine learning models that predict soil behavior in seismic events. Their method stands out as it moves beyond traditional soil testing, which is often limited in scope and comprehensiveness. Instead, the models offer a broader understanding of soil stability, identifying locations suitable for construction and enhancing disaster preparedness. In their study published on October 8, 2024, in ‘Smart Cities,’ the researchers utilized geological data to establish a systematic approach for predicting the depth of bearing layers—an essential measure of soil stability. “This study establishes a high-precision prediction method for unknown points and areas, demonstrating the significant potential of machine learning in geotechnical engineering. These improved prediction models facilitate safer and more efficient infrastructure planning, which is critical for earthquake-prone regions, ultimately contributing to the development of safer and smarter cities,” states Professor Inazumi. The established model incorporated data from standard penetration tests and mini-ram sounding tests to train the ANN in order to predict the soil bearing depth accurately. Applying the bagging technique, which trains the model multiple times on varying data subsets, yielded a remarkable 20 percent increase in prediction accuracy. The resulting contour maps serve as instrumental resources for civil engineers and disaster management professionals, allowing for better identification of stable construction sites and the assessment of earthquake vulnerability in urban landscapes. The researchers aspire to position their machine learning approach as a fundamental component for smart city evolution, emphasizing data-centric strategies for effective urban and infrastructure development. “This study provides a foundation for safer, more efficient, and cost-effective urban development. By integrating advanced AI models into geotechnical analysis, smart cities can better mitigate liquefaction risks and strengthen overall urban resilience,” remarks Professor Inazumi, expressing optimism towards future developments in this field. As they advance, the research team aims to refine their predictive model further by incorporating additional geological factors and tailored models for both coastal and inland regions, acknowledging the impact of groundwater on liquefaction susceptibility.
Liquefaction is a critical risk in urban development, especially in earthquake-prone regions like Japan, where the stability of soil is paramount for infrastructure. The challenge of predicting how soils will behave during seismic activities necessitates advanced technological solutions. This research employs machine learning techniques to create comprehensive soil maps that aid in the identification of stable sites for construction while enhancing disaster preparedness strategies to mitigate potential risks associated with liquefaction during earthquakes.
The implementation of machine learning models for predicting soil stability marks a significant advancement in urban resilience against liquefaction. By providing city planners with detailed soil contour maps, the research contributes to informed infrastructure development, ultimately fostering safer urban environments. As cities continue to grow in the face of natural disasters, employing innovative technologies such as these is crucial for enhancing disaster management and urban planning efforts.
Original Source: www.preventionweb.net