Advancements in Machine Learning for Enhanced Resilience Against Liquefaction in Earthquake-Prone Areas
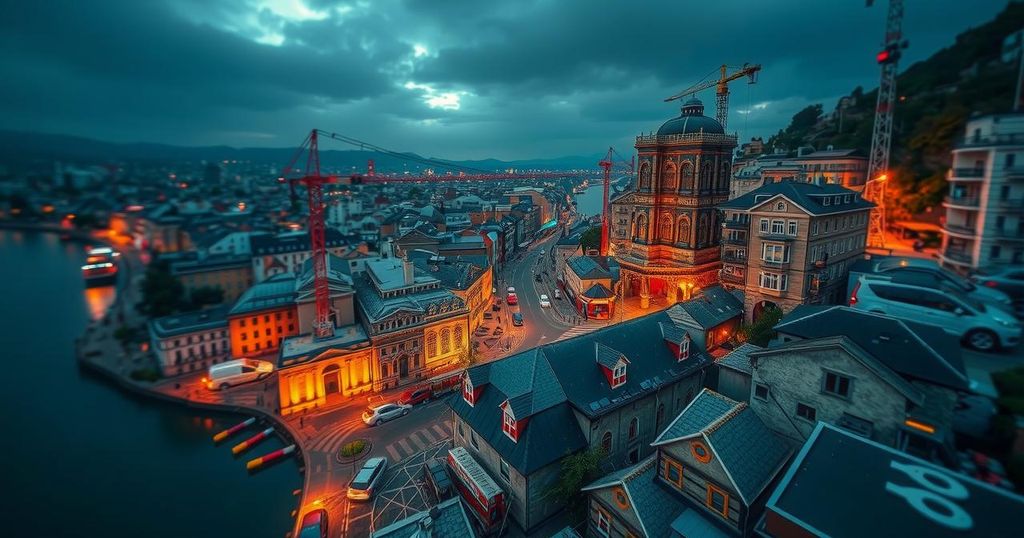
Researchers at Shibaura Institute of Technology have developed machine learning models to predict soil liquefaction during earthquakes. Their study utilizes artificial neural networks to create 3D soil maps, enhancing risk assessment and infrastructure planning in earthquake-prone regions, particularly in Japan. The models demonstrated a 20% improvement in prediction accuracy and aim to facilitate safer urban development.
In response to the growing threat of natural disasters in urban environments, particularly in earthquake-prone regions such as Japan, researchers at Shibaura Institute of Technology are advancing the use of machine learning to enhance infrastructure resilience against liquefaction. Liquefaction is a critical risk during earthquakes, where intense shaking causes saturated, loose soils to behave like liquid, resulting in significant structural damage, such as building settlement and the failure of essential utilities. Historical events like the 2011 Tōhoku earthquake and the more recent Noto earthquake in 2024 underscore the dire implications of this phenomenon, which can devastate urban infrastructure and pose considerable challenges for city planners. To address this pressing issue, Professor Shinya Inazumi and his student Yuxin Cong have developed predictive machine learning models that analyze geological data to assess soil stability during seismic events. Their innovative research, recently published in _Smart Cities_, leverages artificial neural networks (ANNs) and advanced ensemble learning techniques to accurately estimate the depth of bearing soil layers, a vital factor in determining the risk of liquefaction. This method offers a comprehensive and high-resolution analysis of soil conditions, greatly surpassing traditional, localized soil testing methods. In their study, the researchers deployed data from 433 measurement points throughout Setagaya-ku in Tokyo, utilizing standard penetration tests combined with mini-ram sounding tests to ascertain bearing depth and other geospatial factors. By training the ANN on this dataset, the team evaluated its predictive capabilities against actual measurements, achieving a notable 20% accuracy improvement through techniques like bagging (bootstrap aggregation). The resulting contour maps depicting bearing layer depths will serve as essential tools for civil engineers and disaster management professionals. These maps identify optimal construction locations with stable soil and highlight zones susceptible to liquefaction, thereby facilitating improved risk assessment strategies. Professor Inazumi stated, “This study establishes a high-precision prediction method for unknown points and areas, demonstrating the significant potential of machine learning in geotechnical engineering.” Furthermore, the research team envisions expanding their predictive model by integrating additional geological data and customizing analyses for different environments, including coastal regions where groundwater presence is a critical concern. This research represents a significant step toward data-driven urban planning methodologies, aiming to create safer and smarter cities that can withstand the challenges of natural disasters.
The problem of soil liquefaction has become an urgent concern in urban planning, especially in regions prone to earthquakes. Liquefaction occurs when saturated soil loses strength due to seismic activity, essentially causing the ground underneath structures to act like a liquid. This can lead to catastrophic consequences for buildings and infrastructure, as evidenced by past earthquakes in Japan, Canada, and New Zealand. The development of machine learning algorithms to predict soil behavior during such events presents a promising solution to mitigate these risks and enhance infrastructure resilience.
In summary, the integration of machine learning into geotechnical engineering signifies a pivotal advancement in addressing the challenges posed by soil liquefaction in earthquake-prone areas. The research conducted by Professor Inazumi and his team not only enhances the predictive accuracy concerning soil stability but also aids in urban planning and disaster management efforts. By leveraging data-driven approaches, municipalities can create more secure infrastructures, ultimately fostering safer living environments in regions vulnerable to seismic activity.
Original Source: techxplore.com